Contents
- 1 Exploring the Impact of Deep Learning on Modern Technology
- 1.1 Introduction to Deep Learning
- 1.2 Evolution of Deep Learning Technologies
- 1.3 Applications of Deep Learning in Various Industries
- 1.4 Benefits and Challenges of Deep Learning
- 1.5 The Role of Big Data in Deep Learning Advancement
- 1.6 Future Trends in Deep Learning and Technology Integration
- 1.7 Ethical Considerations in Deep Learning Implementation
- 1.8 Conclusion: The Transformative Power of Deep Learning
- 1.9 Frequently Asked Questions (FAQ)
In recent years, deep learning has emerged as a transformative force within the realm of modern technology, reshaping industries and redefining the way we interact with machines. As a subset of artificial intelligence, deep learning leverages complex neural networks to process vast amounts of data, enabling unprecedented levels of accuracy and efficiency in tasks ranging from image recognition to natural language processing.
This article delves into the profound impact of deep learning on various sectors, exploring its evolution, applications, and the challenges it presents. By examining the interplay between deep learning and technological advancements, we aim to provide a comprehensive understanding of how this revolutionary approach is not only influencing current practices but also paving the way for future innovations.
Exploring the Impact of Deep Learning on Modern Technology
Introduction to Deep Learning
What is Deep Learning?
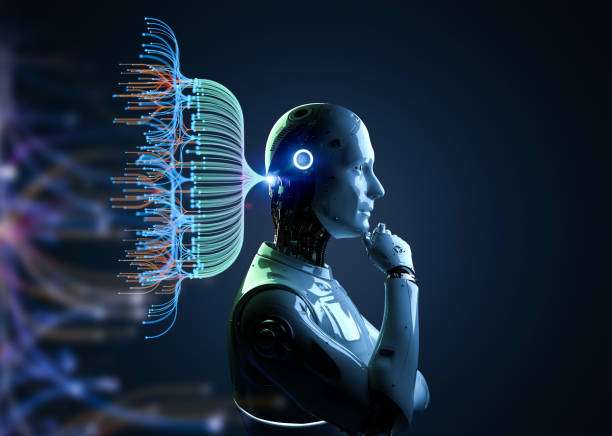
Deep learning is like the rockstar of the artificial intelligence (AI) world—loud, flashy, and capable of jaw-dropping performances. At its core, deep learning is a subset of machine learning that uses algorithms inspired by the structure and function of the human brain, known as artificial neural networks. These networks learn from vast amounts of data, identifying patterns and making predictions that can make even the most skeptical data analyst raise an eyebrow. Whether it’s recognizing your voice when you ask your phone for the weather or helping Facebook tag your friends in photos, deep learning is the magic sauce making it all happen.
Historical Context and Development
While deep learning might feel like the new kid on the tech block, it has roots that dig deep into the soil of computer science history. The concept of neural networks dates back to the 1950s, but it wasn’t until the 2000s that deep learning began to really shine—thanks to increased computing power and the explosion of data generated by the digital age. Early progress was stymied by limitations in hardware and data, but things took a turn in 2012 when a neural network defeated all expectations in the ImageNet competition. Suddenly, researchers paid attention, investment flowed, and deep learning became the belle of the ball, leading to breakthroughs we’re still buzzing about today.
Evolution of Deep Learning Technologies
Key Milestones in Deep Learning
Think of deep learning evolution as a thrilling roller coaster ride, with plenty of ups, downs, and loop-the-loops. Significant milestones have propelled deep learning into the limelight. From the introduction of the first convolutional neural networks (CNNs) for image processing to the development of recurrent neural networks (RNNs) for sequence data, each milestone has helped push the boundaries of what’s possible. The launch of frameworks like TensorFlow and PyTorch democratized access for developers, allowing even the newest coders to get in on the deep learning fun. And let’s not forget the creation of groundbreaking models like OpenAI’s GPT series, which made conversational AI sound more human than ever.
Advancements in Neural Network Architectures
As if designing neural networks wasn’t already a puzzle, researchers have continuously innovated new architectures that take deep learning to dizzying heights. We’ve seen the rise of transformer models that made stage dives into natural language processing, proving that attention isn’t just a human trait, but also a game-changer for machines. Each new architecture, from generative adversarial networks (GANs) to attention mechanisms, has opened new horizons, making deep learning faster and more efficient. It’s like a never-ending game of Tetris, where each piece fits perfectly into the grand design of AI advancement.
Applications of Deep Learning in Various Industries
Healthcare: Revolutionizing Diagnosis and Treatment
In the world of healthcare, deep learning is like having a super-smart assistant on speed dial. It’s revolutionizing everything from diagnosing diseases based on medical images to personalizing treatment plans. Algorithms can sift through medical records and identify patterns that even the most seasoned doctor might miss. Imagine a world where AI helps detect cancers in their earliest stages or predicts patient outcomes with uncanny accuracy. Thanks to deep learning, that future is already beginning to unwrap.
Finance: Enhancing Risk Management and Fraud Detection
In finance, deep learning is like the diligent accountant who never sleeps. It’s continuously analyzing transactions, assessing risk, and sniffing out fraud faster than you can say “money laundering.” By recognizing unusual patterns in transaction data, deep learning models can flag potential fraudulent activity, helping banks protect themselves and their customers. Plus, these technologies are also refining risk assessment models, allowing financial institutions to make more informed investment decisions.
Transportation: Autonomous Vehicles and Beyond
When it comes to transportation, deep learning is driving innovation (pun very much intended). Autonomous vehicles are perhaps the most visible application, where deep learning enables cars to recognize objects, interpret their surroundings, and make split-second decisions. But the impact doesn’t stop there—traffic management systems and logistic optimizations are leveraging deep learning to ensure that your pizza arrives hot and on time, all while navigating the unpredictable chaos of city streets.
Retail: Personalization and Customer Insights
Retailers are using deep learning like a crystal ball that not only predicts what you want but also nudges you toward those impulse buys you didn’t even know you needed. From personalized product recommendations to dynamic pricing strategies, deep learning helps businesses deliver a shopping experience that feels uniquely tailored to each customer. By analyzing purchasing patterns and consumer sentiment, retailers can better anticipate trends and respond to customer needs in real-time.
Benefits and Challenges of Deep Learning
Advantages of Deep Learning Technologies
Deep learning isn’t just a pretty face—it’s packed with benefits that are transforming industries across the board. One of its biggest strengths is its ability to process massive amounts of data quickly and accurately, making it ideal for handling tasks that would take humans ages. The automation of complex processes leads to increased efficiency and productivity, not to mention potentially reducing human error. Plus, the ability to learn continuously means that systems can adapt and improve over time, making deep learning a future-ready investment for companies willing to embrace its power.
Common Challenges and Limitations
Of course, every superhero has its kryptonite, and deep learning is no exception. One of the primary challenges is the need for large datasets, which can be difficult to obtain and manage. Moreover, deep learning models are often seen as “black boxes”—they can deliver remarkable results, but understanding how they arrive at those conclusions can be like deciphering hieroglyphics. Concerns also arise around biases in data that can perpetuate inequalities if not properly addressed. Lastly, the computational power required can be a barrier, making deep learning more accessible to larger organizations while leaving smaller players in the dust.
In the ever-evolving dance of technology, deep learning is a show-stealer—bringing innovation, efficiency, and a touch of glamour to the mundane. Now, let’s follow this thread into the future and see where it leads us!# Exploring the Impact of Deep Learning on Modern Technology
The Role of Big Data in Deep Learning Advancement
Data Requirements for Effective Deep Learning
Deep learning models are like high-performance sports cars—they need a lot of fuel (data) to show their true potential. These models thrive on massive amounts of diverse, high-quality data, as it allows them to learn and recognize patterns efficiently. Imagine teaching a child to identify animals: it helps if you show them thousands of pictures of dogs, cats, and elephants rather than just a couple of each. Similarly, deep learning requires vast datasets to be trained effectively, which is where the delightful world of big data struts onto the stage.
Big Data Technologies Supporting Deep Learning
Now that we’ve established that deep learning loves big data, let’s talk about the tech that makes this love story possible. Frameworks like Apache Hadoop and Apache Spark are like the delivery trucks bringing heaps of data to the deep learning party. They allow for distributed data processing and real-time analytics, ensuring that our models are trained quickly and efficiently. Coupled with tools like TensorFlow and PyTorch, deep learning harnesses the power of big data technologies to innovate at lightning speed. This high-tech fusion fuels advancements across industries, from healthcare to finance, proving that when data and deep learning unite, the results can be nothing short of magical.
Future Trends in Deep Learning and Technology Integration
Emerging Technologies and Innovations
As we peer into the crystal ball of deep learning, it’s clear that new technologies are sprouting up everywhere—think of them as the fresh veggies in your tech salad. From quantum computing to edge AI, the possibilities are boundless. Quantum computing, in particular, holds the promise of processing vast amounts of data at unprecedented speeds, which could revolutionize deep learning capabilities. Meanwhile, innovations in natural language processing (NLP) make it possible for machines to chat with us more like humans. The result? A tech landscape that’s continuously evolving, with deep learning at its heart.
Predictions for Deep Learning’s Role in Future Developments
So, what does the future hold for deep learning? Buckle up, because it looks thrilling! Expect to see deep learning powering hyper-personalized experiences—think Netflix recommendations that feel eerily accurate, or virtual assistants that know you better than your best friend (no pressure, virtual buddy!). Moreover, as industries integrate deep learning into their operations, we’ll likely witness a surge in automation, leading to the need for new skills and roles. The takeaway? Deep learning will not only redefine our technological landscape but will also reshape the very fabric of our daily lives.
Ethical Considerations in Deep Learning Implementation
Bias and Fairness in Deep Learning Models
As powerful as deep learning can be, it’s not without its pitfalls. One of the most pressing concerns is bias—yes, that sneaky little gremlin that can creep into algorithms. If the data used to train a model is biased, the outcomes will be too. This could lead to unfair treatment in applications like hiring, lending, and law enforcement. It’s crucial for researchers and developers to be vigilant, ensuring their models promote fairness and are trained with diverse datasets. After all, nobody wants to be the tech villain in this story.
Privacy Concerns and Data Security
In the realm of deep learning, data is king, but with great power comes great responsibility (thanks, Spider-Man!). The sheer amount of personal data required for effective models raises significant privacy concerns. Data breaches or misuse can lead to catastrophic consequences, sparking a demand for robust security measures. Organizations must prioritize data protection, transparency, and user consent, helping users feel safe while benefiting from the advancements deep learning has to offer. A balance must be struck between innovation and ethics, ensuring that technology serves humanity rather than the other way around.
Conclusion: The Transformative Power of Deep Learning
Summary of Key Insights
In summary, deep learning’s impact on modern technology is both profound and far-reaching. From the importance of big data in fueling these algorithms to the exciting future trends we can expect, deep learning is a driving force behind many innovations today. However, we must tread carefully, navigating the ethical challenges to ensure a fair and secure digital landscape for all.
Final Thoughts on the Future of Deep Learning
The deep learning revolution is a significant technological advancement that is transforming various industries and enhancing our daily lives. However, it also presents challenges, particularly regarding ethics and data security. Despite these challenges, deep learning offers numerous benefits such as improved efficiency and accuracy. As we look towards the future, the evolution of deep learning promises to unlock new possibilities and solutions that can shape our world. By embracing its potential and addressing its challenges, we can harness the transformative power of deep learning to create a better, more connected future. By embracing the unknown and welcoming the tech wonders that lie ahead, we can create a brighter, smarter future.
Frequently Asked Questions (FAQ)
What is the difference between deep learning and traditional machine learning?
Deep learning is a subset of machine learning that uses artificial neural networks with multiple layers (hence “deep”) to process data. Unlike traditional machine learning, which often relies on feature extraction and manual data processing, deep learning automates these processes, allowing it to analyze unstructured data more effectively, such as images and text.
What industries are most impacted by deep learning?
Deep learning is making significant strides in various industries, including healthcare, finance, automotive, retail, and entertainment. In healthcare, it aids in disease diagnosis; in finance, it enhances fraud detection; in automotive, it powers autonomous vehicles; and in retail, it improves customer personalization.
What are the ethical concerns associated with deep learning?
Ethical concerns regarding deep learning primarily include issues of bias, fairness, and privacy. Models can inadvertently learn and propagate biases present in training data, leading to unfair outcomes. Additionally, the use of personal data for training can raise privacy and security concerns that need to be addressed.
How will deep learning evolve in the future?
As technology advances, deep learning is expected to evolve with improvements in algorithms, increased computational power, and better access to big data. Future trends may include enhanced interpretability of models, more efficient training methods, and deeper integration with other emerging technologies, such as quantum computing and edge computing.